‘Accelerating Deep Learning Training Through Transparent Storage Tiering’ is the title of the new paper presented at the 22nd IEEE International Symposium on Cluster, Cloud and Internet Computing (CCGrid 22).
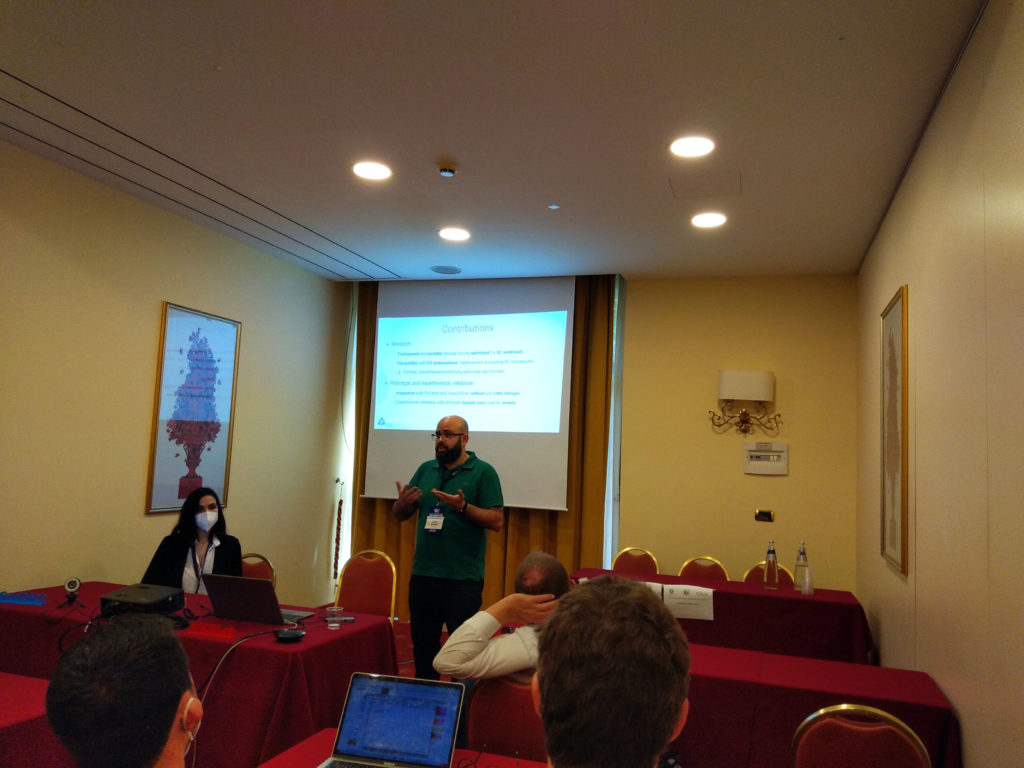
This paper was made by Marco Dantas, Ricardo Macedo, and João Paulo (from INESC TEC), Peter Cui, and Weija Xu (from TACC and UT Austin), and Xinlian Liu (from Hood College).
The paper presents Monarch, a software designed to accelerate the Deep Learning training process in HPC centers. This is achieved by using storage resources that can be found in the compute nodes of many supercomputers, which are often neglected by users. Furthermore, Deep Learning jobs are particularly problematic when they use the supercomputer’s shared storage infrastructures as the main data source. In such a scenario, these jobs contribute to the saturation of these systems.
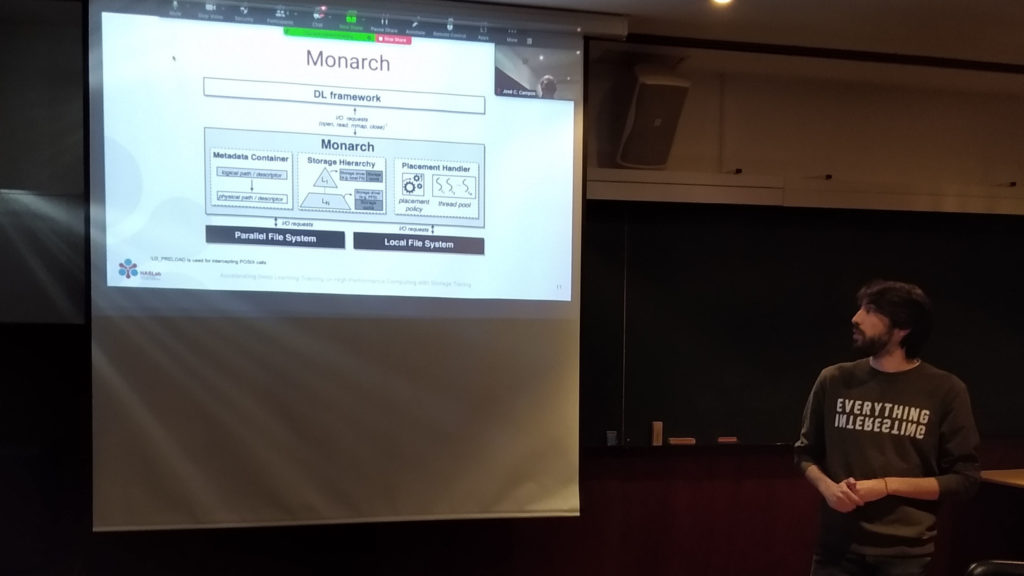
“Monarch, however, is able to reduce that impact, contributing to the Quality of Service (QoS) of all users that also resort to those storage systems”, stated Marco Dantas, one of the authors.
It is important to mention that Monarch is the main result of the Master thesis developed by Marco Dantas, which was supervised by João Paulo, Ricardo Macedo, and Rui Oliveira, INESC TEC researchers, and which was graded with 20 values. The defense took place on May 23, at the University of Minho.
Read the paper here.